Multimorbidity in autistic people and people with learning disabilities
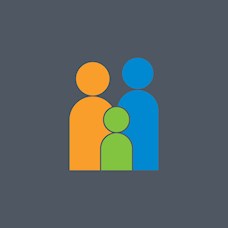
Background
A multimorbidity index is a list of conditions that assigns a score to a patient based on the conditions they have. This score is then used to predict deterioration in the patient. There is a large variety of multimorbidity indices available, but all are based on information from the general population. Research has shown that the physical and mental health conditions commonly experienced by autistic people or people with learning disabilities are different from those in the general population. In addition, general health, multimorbidity and premature mortality have been shown to be worse in autistic people and people with learning disabilities as compared to the general population.
What we did
Using data from the British Heart Foundation COVID consortium spanning the whole population of England, we used machine learning techniques to create a multimorbidity index for autistic people based on autistic people only. We then looked at how well this multimorbidity index predicted COVID-19 mortality in autistic people when compared with a multimorbidity index based on the general population.
We investigated what the differences are between patterns of physical and mental health conditions experienced by autistic people and people with learning disabilities as compared to the general population. Based on these differences, we aim to determine whether there is a need for adjustment of specific tools, such as a multimorbidity index to predict patient deterioration, for autistic people and people with learning disabilities.
What we found
The index based on autistic people only was slightly better at predicting COVID-19 mortality in autistic people. Because our data had few people, this suggests that a larger sample or one that looks at mortality beyond COVID-19 may have more profound differences between predictions. Our study shows that there is a case to be made to create multimorbidity indices specifically for autistic people to improve their accuracy.
Key findings:
• A tailored index performs better than general-population based tools. Even though the difference is small, when it comes to mortality this can make a big difference.
• Since this study was a case-in-point study, its scope is quite narrow. Despite that, we were able to find a better performance for the tailored index. It is reasonable to assume that replication in more generalised mortality may produce more significant findings. This study contributes to the body of evidence suggesting that precision medicine is especially important in marginalised groups.
• Further, it can help in identifying conditions that are currently under-explored but important for autistic adults. In our study, thyroid and cardiac conditions were especially significant in predicting COVID-19 related mortality in autistic adults. Yet, even outside of COVID-19 context these conditions are understudied in autism.
What these findings mean
Precision medicine/healthcare has become increasingly recognised as important in clinical decision making. The ASD-MI is one of many tools that illustrates the potential for tailored risk tools.
Our data highlights the importance of understanding the differing health profiles of autistic adults, highlighted by the improved accuracy of the ASD-MI and emergence of understudied conditions as important mortality predictors.
This index may have value for:
- GPs or hospitals: those that make use of risk predicting tools (e.g., the golden standard Charlson Index or the more updated Quan Index).
- NHS Digital, HER system developers: those that integrate risk models into existing healthcare databases.
Researchers working with health data or risk prediction, autism, medical education. - Advocacy groups: those that might want need evidence to highlight the importance of precision medicine or the existence of different health patterns in autistic people.
A pre-print version of this research paper is currently available at https://www.medrxiv.org/content/10.1101/2024.02.23.24303273v1
For further information on this research, please contact Dewy Nijhof
An easy read version of the summary is available here.
Page updated 19th May 2025.